OpenSOC
OpenSOC was created by Cisco to attack the “big data problem” for their Managed Threat Defense offering. Cisco has developed a fully managed service delivered by Cisco Security Solutions to help customers protect against known intrusions, zero-day attacks, and advanced persistent threats. Cisco has a global network of security operations centers (SOCs) ensuring constant awareness and on-demand analysis 24 hours a day, 7 days a week. They needed the ability to capture full packet-level data and extract protocol metadata to create a unique profile of customer’s network and monitor them against Cisco threat intelligence. As you can imagine, performing big data analytics for one organization is a challenge, Cisco has to perform big data analytics for numerous customers including very large enterprises. The goal with OpenSOC is to have a robust framework based on proven technologies to combine machine learning algorithms and predictive analytics to detect today’s security threats.
The following are some of the benefits of OpenSOC:
- The ability to capture raw network packets, store those packets, and perform traffic reconstruction
- Collect any network telemetry, perform enrichment, and generate real-time rules-based alerts
- Perform real-time search and cross-telemetry matching
- Automated reports
- Anomaly detection and alerting
- Integration with existing analytics tools
The primary components of OpenSOC include the following:
- Hadoop
- Flume
- Kafka
- Storm
- Hive
- Elasticsearch
- HBase
- Third-party analytic tool support (R, Python-based tools, Power Pivot, Tableau, and so on)
The sections that follow cover these components in more detail.
Hadoop
The Apache Hadoop or “Hadoop” is a project supported and maintained by the Apache Software Foundation. Hadoop is a software library designed for distributed processing of large data sets across clusters of computers. One of the advantages of Hadoop is its ability to using simple programming models to perform big data processing. Hadoop can scale from a single server instance to thousands of servers. Each Hadoop server or node performs local computation and storage. Cisco uses Hadoop clusters in OpenSOC to process large amounts of network data for their customers, as part of the Managed Threat Defense solution, and it also uses Hadoop for its internal threat intelligence ecosystem.
Hadoop includes the following modules:
- Hadoop Common: The underlying utilities that support the other Hadoop modules.
- Hadoop Distributed File System (HDFS): A highly scalable and distributed file system.
- Hadoop YARN: A framework design for job scheduling and cluster resource management.
- Hadoop MapReduce (MapR): A system designed for parallel processing of large data sets based on YARN.
Figure 5-2 illustrates a Hadoop cluster.
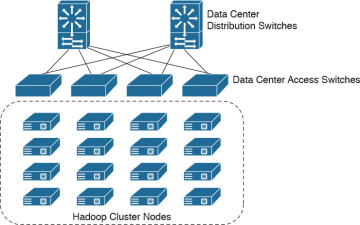
Figure 5-2 Hadoop Cluster Example
In Figure 5-2, a total of 16 servers are configured in a Hadoop cluster and connected to the data center access switches for big data processing.
HDFS
HDFS is a highly scalable and distributed file system that can scale to thousands of cluster nodes, millions of files, and petabytes of data. HDFS is optimized for batch processing where data locations are exposed to allow computations to take place where the data resides. HDFS provides a single namespace for the entire cluster to allow for data coherency in a write-once, read-many access model. In other words, clients can only append to existing files in the node. In HDFS, files are separated into blocks, which are typically 64 MB in size and are replicated in multiple data nodes. Clients access data directly from data nodes. Figure 5-3 shows a high-level overview of the HDFS architecture.
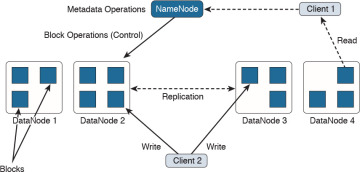
Figure 5-3 HDFS Architecture
In Figure 5-3, the NameNode (or Namespace Node) maps a filename to a set of blocks and the blocks to the data nodes where the block resides. There are a total of four data nodes, each with a set of data blocks. The NameNode performs cluster configuration management and controls the replication engine for blocks throughout the cluster. The NameNode metadata includes the following:
- The list of files
- List of blocks for each file
- List of data nodes for each block
- File attributes such as creation time and replication factor
The NameNode also maintains a transaction log that records file creations, deletions, and modifications.
Each DataNode includes a block server that stores data in the local file system, stores metadata of a block, and provisions data and metadata to the clients. DataNodes also periodically send a report of all existing blocks to the NameNode and forward data to other specified DataNodes as needed. DataNodes send a heartbeat message to the NameNode on a periodic basis (every 3 seconds by default), and the NameNode uses these heartbeats to detect any DataNode failures. Clients can read or write data to each data block, as shown in Figure 5-3.
Flume
OpenSOC uses Flume for collecting, aggregating, and moving large amounts of network telemetry data (like NetFlow, syslog, SNMP, and so on) from many different sources to a centralized data store. Flume is also licensed under the Apache license. Figure 5-4 shows how different network telemetry sources are sent to Flume agents for processing.

Figure 5-4 Network Telemetry Sources and Flume
Flume has the following components and concepts:
- Event: A specific unit of data that is transferred by Flume, such as a single NetFlow record.
- Source: The source of the data. These sources are either actively queried for new data or they can passively wait for data to be delivered to them. The source of this data can be NetFlow collectors, server logs from Splunk, or similar entities.
- Sink: Delivers the data to a specific destination.
- Channel: The conduit between the source and the sink.
- Agent: A Java virtual machine running Flume that comprises a group of sources, sinks, and channels.
- Client: Creates and transmits the event to the source operating within the agent.
Figure 5-5 illustrates Flume’s high-level architecture and its components.

Figure 5-5 Flume Architecture
Kafka
OpenSOC uses Kafka as its messaging system. Kafka is a distributed messaging system that is partitioned and replicated. Kafka uses the concept of topics. Topics are feeds of messages in specific categories. For example, Kafka can take raw packet captures and telemetry information from Flume (after processing NetFlow, syslog, SNMP, or any other telemetry data), as shown in Figure 5-6.
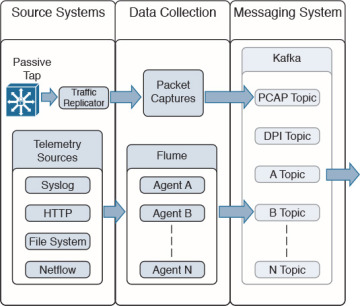
Figure 5-6 Kafka Example in OpenSOC
In Figure 5-6, a topic is a category or feed name to which log messages and telemetry information are exchanged (published). Each topic is an ordered, immutable sequence of messages that is continually appended to a commit log.
Kafka provides a single “consumer” abstraction layer, as illustrated in Figure 5-7.

Figure 5-7 Kafka Cluster and Consumers
Consumers are organized in consumer groups, and each message published to a topic is sent to one consumer instance within each subscribing consumer group.
All consumer instances that belong to the same consumer group are processed in a traditional queue load balancing. Consumers in different groups process messages in a publish-subscribe mode, where all the messages are broadcast to all consumers.
In Figure 5-7, the Kafka cluster contains two servers (Server 1 and Server 2), each with two different partitions. Server 1 contains partition 0 (P0) and partition 1 (P1). Server 2 contains partition 2 (P2) and partition 3 (P3). Two consumer groups are illustrated. Consumer Group 1 contains consumers A, B, and C. Consumer Group 2 contains consumers: D and E.
Kafka provides parallelism to provide ordering guarantees and load balancing over a pool of consumer processes. However, there cannot be more consumer instances than partitions.
Storm
Storm is an open source, distributed, real-time computation system under the Apache license. It provides real-time processing and can be used with any programming language.
Hadoop consists of two major components: HDFS and MapReduce. The early implementations of Hadoop and MapReduce were designed on batch analytics, which does not provide any real-time processing. In SOCs, you often cannot process data in batches, and so it can take several hours to complete the analysis.
OpenSOC uses Storm because it provides real-time streaming and because of its amazing ability to process big data, at scale, in real time. Storm can process data at over a million tuples processed per second per node. Figure 5-8 shows how Kafka topics feed information to Storm to provide real-time processing.
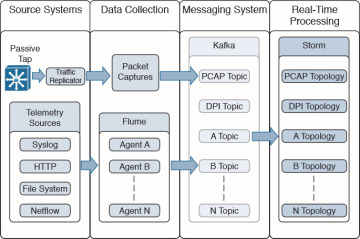
Figure 5-8 Storm in OpenSOC
Hive
Hive is a data warehouse infrastructure that provides data summarization and ad hoc querying. Hive is also a project under the Apache license. OpenSOC uses Hive because of its querying capabilities. Hive provides a mechanism to query data using a SQL-like language that is called HiveQL. In the case of batch processing, Hive allows MapR programmers use their own custom mappers.
Figure 5-9 shows how Storm feeds into Hive to provide data summarization and querying.
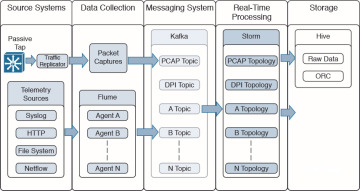
Figure 5-9 Hive in OpenSOC
Storm can also feed into HBase and Elasticsearch. These are covered in the following sections.
Elasticsearch
Elasticsearch is a scalable and real-time search and analytics engine that is also used by OpenSOC. Elasticsearch has a very strong set of application programming interfaces (APIs) and query domain-specific languages (DSLs). It provides full query DSL based on JSON to define such queries. Figure 5-10 shows how Storm feeds into Elasticsearch to provide real-time indexing and querying.
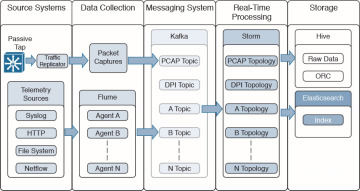
Figure 5-10 Elasticsearch in OpenSOC
HBase
HBase is scalable and distributed database that supports structured data storage for large tables. You guessed right: HBase is also under the Apache license! OpenSOC uses HBase because it provides random and real-time read/write access large data sets.
HBase provides linear and modular scalability with consistent database reads and writes.
It also provides automatic and configurable high-availability (failover) support between Region Servers. HBase is a type of “NoSQL” database that can be scaled by adding Region Servers that are hosted on separate servers.
Figure 5-11 shows how Storm feeds into HBase to provide real-time indexing and querying.
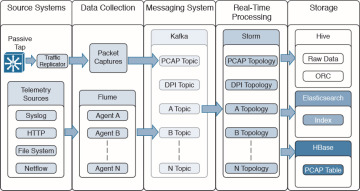
Figure 5-11 HBase in OpenSOC
Third-Party Analytic Tools
OpenSOC supports several third-party analytic tools such as:
- R-based and Python-based tools
- Power Pivot
- Tableau
Figure 5-12 shows the complete OpenSOC architecture, including analytics tools and web services for additional search, visualizations, and packet capture (PCAP) reconstruction.

Figure 5-12 OpenSOC Architecture
Other Big Data Projects in the Industry
There are other Hadoop-related projects used in the industry for processing and visualizing big data. The following are a few examples:
- Ambari: A web-based tool and dashboard for provisioning, managing, and monitoring Apache Hadoop clusters.
- Avro: A data serialization system.
- Cassandra: A scalable multimaster database with no single points of failure.
- Chukwa: A data collection system for managing large distributed systems.
- Mahout: A scalable machine learning and data mining library.
- Pig: A high-level data-flow language and execution framework for parallel computation.
- Spark: A fast and general compute engine for Hadoop data.
- Tez: A generalized data-flow programming framework, built on Hadoop YARN.
- ZooKeeper: A high-performance coordination service for distributed applications.
Berkeley Data Analytics Stack (BDAS): A framework created by Berkeley’s AMPLabs. BDAS has a three-dimensional approach: algorithms, machines, and people. The following are the primary components of BDAS:
- Akaros: An operating system for many-core architectures and large-scale SMP systems
- GraphX: A large-scale graph analytics
- Mesos: Dynamic resource sharing for clusters
- MLbase: Distributed machine learning made easy
- PIQL: Scale independent query processing
- Shark: Scalable rich analytics SQL engine for Hadoop
- Spark: Cluster computing framework
- Sparrow: Low-latency scheduling for interactive cluster services
- Tachyon: Reliable file sharing at memory speed across cluster frameworks
You can find detailed information about BDAS and Berkeley’s AMPLabs at https://amplab.cs.berkeley.edu